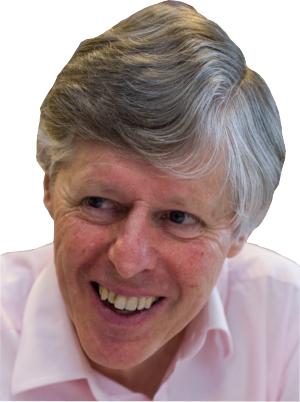
Iain Currie
Dr Iain Currie was an Associate Professor in the School of Mathematical and Computer Sciences at Heriot-Watt University, and a long-term collaborator of the Longevitas team. He sadly passed on 24th May 2022 and is greatly missed.
Articles written by Iain Currie
Back to the future with Whittaker smoothing
Forecasting with penalty functions - Part III
This is the last of my three blogs on forecasting with penalties. I discussed the 1-d case in the first blog and the 2-d case in the second. Here we discuss some of the properties of 2-d forecasting. Some readers may find some of my remarks surprising, even paradoxical.
Forecasting with penalty functions - Part II
Our first blog in this series of three looked at forecasting log mortality with penalties in one dimension, i.e. forecasting with data for a single age. We now look at the same problem, but in two dimensions. Figure 1 shows our data. We see an irregular surface sitting on top of the age-year plane. Just as in the 1-d case, we see an underlying smooth surface, and it is this surface that we wish both to estimate and to forecast.
Forecasting with penalty functions - Part I
There is much to say on the topic of penalty forecasting, so this is the first of three blogs. In this blog we will describe penalty forecasting in one dimension; this will establish the basic ideas. In the second blog we will discuss the case of most interest to actuaries: two-dimensional forecasting. In the final blog we will discuss some of the properties of penalty forecasting in two dimensions.
Simulating the Future
This blog has two aims: first, to describe how we go about simulation in the Projections Toolkit; second, to emphasize the important role a model has in determining the width of the confidence interval of the forecast.
Demography's dark matter: measuring cohort effects
My last blog generated quite a bit of interest so I thought I'd write again on cohorts. It's easy to (a) demonstrate the existence of a cohort effect and to (b) fit models with cohort terms, but not so easy to (c) interpret or forecast the fitted cohort coefficients. In this blog I'll fit the following three models:
Forecasting with cohorts for a mature closed portfolio
At a previous seminar I discussed forecasting with the age-period-cohort (APC) model:
$$ \log \mu_{i,j} = \alpha_i + \kappa_j + \gamma_{j-i}$$
Effective dimension
Actuaries often need to smooth mortality rates. Gompertz (1825) smoothed mortality rates by age and his famous law was a landmark in this area. Figure 1 shows the Gompertz model fitted to CMI assured lives data for ages 20–90 in the year 2002. The Gompertz Law usually breaks down below about age 40 and a more general smooth curve would be appropriate. However, a more general smooth curve would obviously require more parameters than the two for the simple Gompertz model.
Hitting the target, but missing the point
Canonical correlation
At our seminar earlier this year I looked at the validity of assumptions underpinning some stochastic projection models for mortality. I looked at the assumption of parameter independence in forecasting, and examined whether this assumption was borne out by the data. It transpires that the assumption of independence is a workable assumption for some models, but not for others. This has important consequences in a Solvency II context — an internal model must be shown to have assumptions grounded in fact.