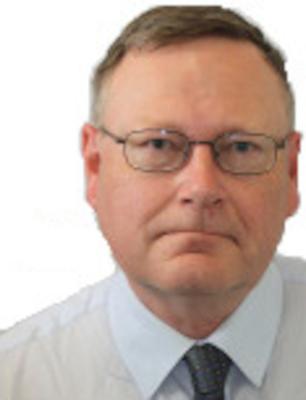
Angus Macdonald
Articles written by Angus Macdonald
More than one kind of information
This collection of blogs is called Information Matrix, and it is named after an important quantity in statistics. If we are fitting a parametric model of the hazard rate, with log-likelihood:
\[ \ell( \alpha_1, \ldots, \alpha_n ) \]
as a function of \(n\) parameters \(\alpha_1, \ldots, \alpha_n\), then the information matrix is the matrix of second-order partial derivatives of \(\ell\). That is, the matrix \({\cal I}\) with \(ij\)th component:
Less is More: when weakness is a strength
A mathematical model that obtains extensive and useful results from the fewest and weakest assumptions possible is a compelling example of the art. A survival model is a case in point. The only material assumption we make is the existence of a hazard rate, \(\mu_{x+t}\), a function of age \(x+t\) such that the probability of death in a short time \(dt\) after age \(x+t\), denoted by \({}_{dt}q_{x+t}\), is:
\[{}_{dt}q_{x+t} = \mu_{x+t}dt + o(dt)\qquad (1)\]
The Karma of Kaplan-Meier
Our new book, Modelling Mortality with Actuarial Applications, describes several non-parametric estimators of two quantities:
Stopping the clock on the Poisson process
"The true nature of the Poisson distribution will become apparent only in connection with the theory of stochastic processes\(\ldots\)"
Feller (1950)
The Curse of Cause of Death Models
Stephen's earlier blog explained the origin of the very useful result relating the life-table survival probability \({}_tp_x\) and the hazard rate \(\mu_{x+t}\), namely:
\[ {}_tp_x = \exp \left( - \int_0^t \mu_{x+s} \, ds \right). \qquad (1) \]
To complete the picture, we add the assumption that the future lifetime of a person now aged \(x\) is a random variable, denoted by \(T_x\), and the connection with expression (1) which is:
Introducing the Product Integral
Of all the actuary's standard formulae derived from the life table, none is more important in survival modelling than:
\[{}_tp_x = \exp\left(-\int_0^t\mu_{s+s}ds\right).\qquad(1)\]
Everything points to Poisson
One recurring theme in our forthcoming book, Modelling Mortality with Actuarial Applications, is the all-pervading role of likelihoods that suggest the lurking presence of a Poisson distribution. A popular assumption in modelling hazard rates is that the number of deaths observed at any given age is a Poisson random variable, so perhaps that might explain it?